Keynote Speakers
Keynote I
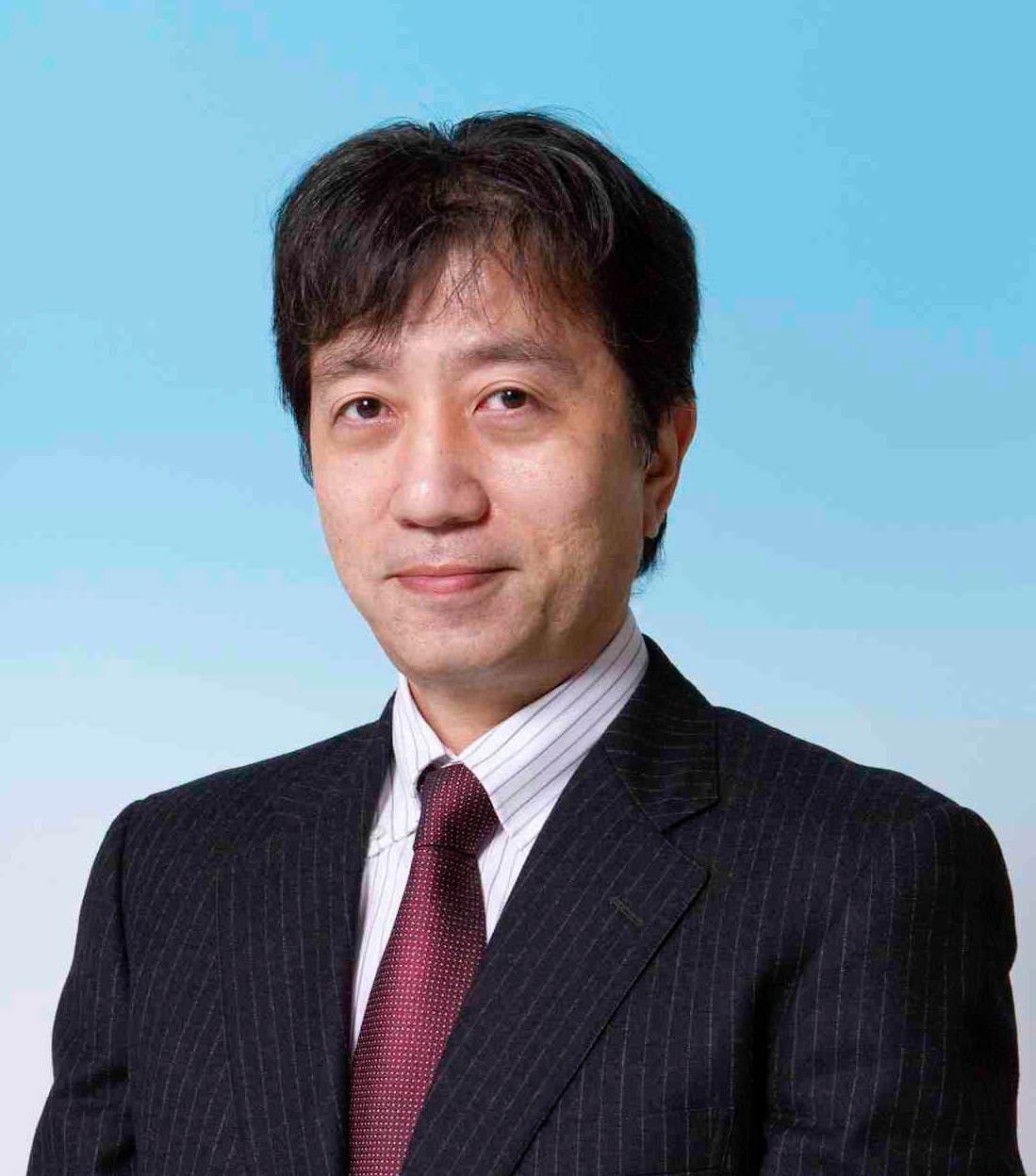
Prof. Masaki Aida, Tokyo Metropolitan University, Japan
Title: Fundamental Model of Online User Dynamics Based on a Causal Framework
User dynamics in online social networks have come to have a great impact not only on online society but also on real life. Therefore, understanding online user dynamics is an important issue. Of course, it is difficult to understand all of the complex online user dynamics, but it may be possible to describe their characteristics in a particular way. This talk introduces an attempt to give a mathematical model of online user dynamics based on a causal framework in which the mutual influences working between users are propagated at finite speeds via an online social network. This model can theoretically explain various phenomena including the intensity of user dynamics diverges, such as online flaming phenomena, and the phenomenon that information propagation is restricted only within a specific community, such as polarization.
Biography
Prof. Masaki Aida received his B.S. degree in Physics and M.S. degree in Atomic Physics from St. Paul's University, Tokyo, Japan, in 1987 and 1989, respectively, and his Ph.D. in Engineering from the University of Tokyo, Japan, in 1999. In April 1989, he joined NTT Laboratories. From April 2005 to March 2007, he was an Associate Professor at the Faculty of Systems Design, Tokyo Metropolitan University. He has been a Professor of the Graduate School of Systems Design, Tokyo Metropolitan University since April 2007. His current interests include analysis of social network dynamics and distributed control of computer communication networks. He received the Best Tutorial Paper Award and the Best Paper Award of IEICE Communications Society in 2013 and 2016, respectively, and IEICE 100-Year Memorial Paper Award in 2017. He is a fellow of IEICE, a senior member of IEEE, and a member of ACM and ORSJ.
Keynote II
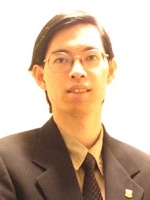
Prof. Carson K. Leung, University of Manitoba, Canada
Title: Big data analytics on COVID-19 epidemiological data
In the current era of big data, high volume of big data can be generated and collected from a wide variety of rich data sources at a rapid rate. Embedded in these big data are useful information and valuable knowledge. Examples include healthcare and epidemiological data such as data related to patients who suffered from viral diseases like the coronavirus disease 2019 (COVID-19). Knowledge discovered from these epidemiological data via data science helps researchers, epidemiologists, and policy makers to get a better understanding of the disease, which may inspire them to come up ways to detect, control and combat the disease. This talk presents big data analytics solutions for analyzing COVID-19 epidemiological data. The solutions help users to get a better understanding of information about COVID-19 cases. Evaluation on real-life COVID-19 data across Canadian provinces show the benefits of big data analytics in discovering useful knowledge from COVID-19 epidemiological data.
Biography
Prof. Carson Leung obtained his BSc(Hons), MSc and PhD all from the University of British Columbia, Canada. He is currently a Professor of Computer Science at the University of Manitoba, Canada. He has contributed more than 300 refereed publications on the topics of big data, bioinformatics, computational intelligence, cognitive computing, data analytics, data mining, data science, fuzzy systems, health informatics, machine learning, social network analysis, and visual analytics. He received Best Paper awards in IEEE DataCom 2021, IEEE SmartData 2021, and IEEE BigComp 2022. He has also served on the Organizing Committee of the ACM CIKM, ACM SIGMOD, IEEE DSAA, IEEE ICDM, and other conferences. He is a Senior Member of the ACM and the IEEE; he is also an IEEE Computer Society Distinguished Contributor.